Â
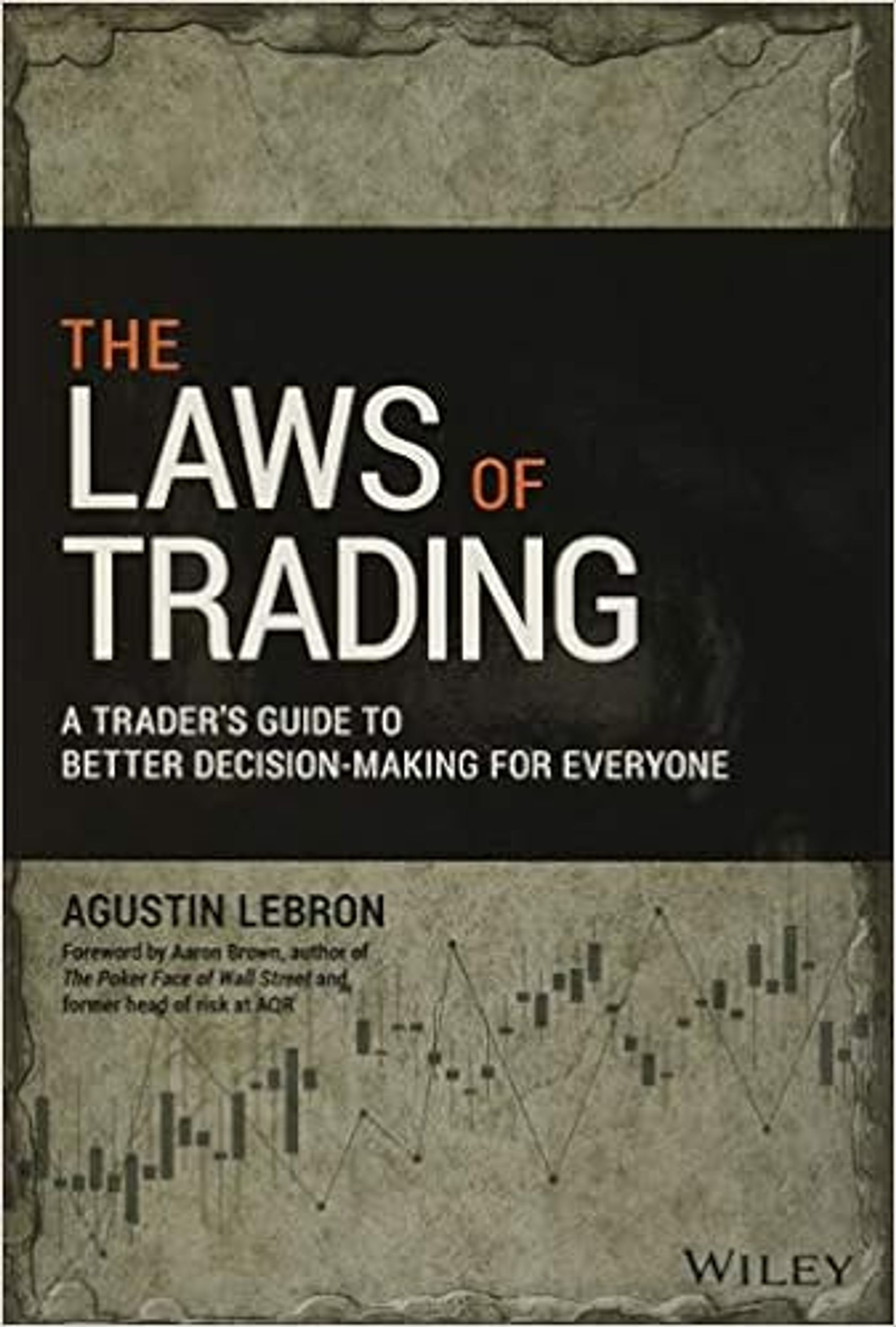
Â
My Introduction
If I ran a trading firm this would be Day 1 reading. After finding out where the bathroom is and filling out your W-4, you would be handed this book and told to finish it by tomorrow.
After 1 year on the job, you are required to re-read it. There are many sentences in this book that serve as somewhat off-hand or connecting, but are deeply insightful. The kinds of things that would not be perceived by a novice but veterans will recognize they are reading something by a deeply experienced professional. As a veteran of options trading, I found this feature makes the book transcend being informative into being delightful.
Trading is the art of decision-making turned into a high-rep game. It requires:
- multi-level thinking
- sound epistemology
- discipline
- self-awareness
- self-honesty
- alignment
- humility
- curiosity
- competitiveness
- collaboration
- creativity
- comparing
Â
It is deeply intertwined with technology, math, and economic reasoning.
This book is an instant classic. The rules in the book are reductions of vast, hard-fought institutional knowledge and the leading edge of thinking about risk.
The author combined his training and experience at Jane Street, a legendary quant trader and market-making firm, with a broad intellectual acumen. Both his engineering background and affinity for liberal arts and philosophy come through to create a guide that transcends a single discipline.
Personally, reading this book left me with nostalgia as the type of thinking was the water I swam in when I was at SIG (Jane Street’s lineage traces to SIG alumni who became under-the-radar legends themselves). My second personal feeling is, “damn, I wish I wrote that book.” Except I couldn’t. The author is an elite synthesizer with a nuanced comprehension for coding, organizational behavior, interviewing, and data analysis.
Â
My notes are below. They are sparse compared to the depth of insight crammed into 250 pages. Every chapter covers a “rule”, situates that rule in the context of finance, then applies it to decisions all people face in the course of life.
Â
Chapter 1: Motivation
Rule: Know why you are trading before you do a trade
Why are you trading? Think it's to make money but is it?
That’s just one reason and it’s less common than you think. Self-awareness would reveal how often the reasons people trade are
- boredom
- desire for a big score
- intellectual validation.
- risk-seeking behavior
- Prison is boring so when criminals get released the opposite of what we want happens...they go searching for their next caper. Alex Honnold doesn't have a death wish but needs risk to feel alive.
- Risk-seeking behavior is robust across a person's domains. Risk setpoints are personal preferences for risk. Poker itch was replaced by trading job for Agustin.
If you motivation is not to make money, you are up against an industry that is and is dialed in on how to extract it from you.
This reminds me of Cedric Chin’s musing on scrub players:
Are You Playing to Play, or Playing to Win?
Staying focused with proper motivation is difficult with willpower.
- Having a set of people with whom you can talk about your trading ideas, creating a systematic process for evaluating them, making plans, and evaluating those plans, all of these are ideas that are fundamentally social and cultural. If you're successful in creating such a culture, the desirable habits become automatic and the day-to-day decision-making inherent in trading becomes the sort of process you want.
SIG director Todd Simkin has also emphasized the importance of culture, teamwork, and communication in keeping yourself focused on the task at hand: making great decisions.
Notes From Todd Simkin On The Knowledge Project
Â
- Use pre-commitments
great examples: being well-researched before buying a car to avoid being upsold or deceived, bad examples could be things like stikk.com that rely on external motivation
Â
Chapter 2: Adverse selection
Rule: You are never happy with the size you traded
- Good trades make you wish you traded bigger, bad trades make you wish you traded less or none. The very act of trading can cause regret.
Winners Curse
Winners curse means any bidding strategy requires the bidder to estimate item's fair value conditional on having won the auction.
This requires estimating how many bidders and how wide their uncertainty is regarding the item. But the problem is that some bidders are better informed than others. So if you are relatively uninformed and win the auction you are sad.
The upshot: specialize and stick to markets you understand well
Special sits trading
- He discusses special situation trading which lends itself to deep, bespoke thinking. It’s not the type of work that is automated, so human discretion and skill can be rewarded. [me: this is why skilled traders who leave the biz focus on special sits vs” normal trading”. Technological disadvantages carry less weight]
A practical example of Bayesian updating
Interpreting a market maker's width as an expression of confidence and using that to update your fair value by weighting their mid price by the confidence.
If I'm 54.10-54.30 and you are 53.50-53.90 then I'm 2x as confident. So my new fair value is 2/3 x 54.2 +1/3 x 53.70 = 54.03
Interviews
- When applying to jobs try to have the most desirable interviews occur later than the less desirable ones so you can practice.
- Interviewers are hand chosen so if you are not impressed by your interviewer you are likely to be disappointed by your new colleagues
Â
Chapter 3: Risk
Rule: Take the risks you are paid to take. Hedge the others.
Â
This chapter is a thorough walk-thru of how trades can be expressed in so many different ways. [Me: Simple buying or selling an asset is a blunt trade expression. Derivatives allow the single summary price of an asset to be decomposed into finer bets. You can bet on distributions and timing in ways that trading the underlyer do not allow.]
[Me: The rule of this chapter was one I regularly employed. Hedge the exposures you don’t want because you have no edge in predicting. Isolate your bets so they map as closely as possible to your reason for wanting the risk.]
Â
Chapter 4: Liquidity
Rule: Put on a risk using the most liquid instrument for that risk
Another great chapter on trade expressions (see examples for FITB). Walks through the costs of putting the trades on and how specific/general the trade expressions are and how you need to have a strong understanding of what you are betting on.
Â
Chapter 5: Edge
Rule: If you can’t explain your edge in 5 minutes, you don’t have a very good one. OR The Long term profitability of an edge is inversely proportional to how long it takes to explain it
Â
This chapter celebrates the importance of the qualitative and stories. The reasoning might surprise those with highly left-brained perspectives on trading .
Â
Chapter 6: Models
Rule: The model expresses the edge
Models need to simplify this world in order to make sense of it, and that simplification must be useful. How do you evaluate usefulness?
The model expresses the edge, not the other way around. A model’s usefulness is only meaningful in the context of the story you want to tell about the trade you want to do. In particular, even if a model makes good predictions about some future value or event that knowledge is useless without also knowing how to take advantage of that prediction. If a model makes good predictions without that model’s predictions being explainable in terms of the edge you claim to have found it is difficult to have confidence in the model’s reliability.
[he gives the example of a good predictive model that doesn’t actually express an edge because you can’t get filled on its signals. You can’t execute either because of competition (others have a similar model and there’s a race) or liquidity. Also reminds me of an interview with venture capitalist Mitchell Green. He lists the 10 criteria his firm uses to prospect investments. He knows they will never find one that checks every box:
If you call 1000 companies, you might find 10 that meet all the criteria. None of them will take your money. They won't take my money. They won't take Sequoia's money, they won’t take GA’s money, nobody's money. And so you're trying to find ones that meet five to seven that are awesome.
The difference between generative and phenomenological models.
I hadn’t heard this distinction labeled as such before. Knowledge of this discussion will raise your awareness of any process you are executing.
- Characteristics of good models specifically robustness and inspectability
- Best practices for using data and model design considerations.
- A callback to chapter 1: We can re-state our first rule “know why you’re doing a trade before you do it” as a rule about mental models about ourselves “develop a good model of your own motivations”. The idea of knowing yourself at least as well as you know anyone else ends up becoming an exhortation to really spend the time to understand yourself, and to build the best models you can about your own nature.
Â
Chapter 7: Costs and Capacity
Rule: If you think your costs are negligible relative to your edge you’re wrong about at least one of them.
4 quadrants of costs
- Cost is either variable (it could be linear or non-linear) or fixed
- A cost is visible vs invisible
[Me: My favorite invisible cost is the fact that every time you trade, you leak information into the world. This is important because it allows others to reverse engineer your ideas. This was also why the trading pits were a great place to learn.]
A Little-Appreciated Fact about Trade Depreciation
All strategies in a perfectly efficient market (again, ignoring other costs) have an expected value of 0
So far, the discussion of trade depreciation has either explicitly or implicitly assumed that you're undertaking profitable trades. But what if you're doing trades you expect to lose money? Recall the discussion on risk in Chapter 3, where you saw that it can be quite rational to lose money hedging in order to achieve a better risk profile for a trade. So how does trade depreciation work with "bad" trades? As it turns out, bad trades get better! Recall that every trade you do is a signal to the world. Your hedging behavior is, over time, reliably signaling "I don't know anything, I'm happy to lose money here. The world will react to this signal by competing to trade against you. This competition is good for your hedging trades: the competition will, in aggregate, provide you with a better price over time and your hedges will lose less money.
In fact, you can restate the insight about trade depreciation more simply: over time the edge of any trade goes to zero (ignoring trading fees, financing cost, etc.). Whether it started on the positive or negative side is not important; over time the world will adapt and markets will become more efficient. And efficient markets, by their very definition, do not admit profitable or unprofitable strategies.
Lessons from Bell Labs
Summarizing, we can identify three main sociological facts about Bell Labs that, if people like Mervin Kelly are to be believed, created the conditions for such a creative workplace:
- Hire the best people possible and create a culture where openness and transmission of ideas are the highest ideal.
- Invest heavily in educating your workforce. Don't outsource the task of designing the curriculum, nor the one of teaching it. • Connect basic research to real-world problems as much as possible. Segmentation and stratification of concerns is a structure to be assiduously avoided.
- Failure is a necessary counterpart to exploratory research. Failed projects do not reflect badly on the project team.
Given all we've been told about disruptive innovation in the last two decades, it's easy to view the above list cynically as a set of platitudes about creating a good work environment. Yet arguably, the reason they seem like platitudes is because the ideas are easy to co-opt. It's straightforward to create the veneer of openness, of education, of practicality, but another thing altogether to actually put these ideas into true practice. And let's not forget, Bell Labs pioneered this sociology of innovation nearly a century before people like Clayton Christensen studied these ideas.
Â
Chapter 8: Possibility
Rule: Just because something has never happened doesn’t mean it can’t. Corollary: Enough people relying on something being true makes it false
Â
- This chapter is philosophical and tackles the anti-inductive nature of markets by reviewing memorable market events often tied to their self-fulfilling (reflexivity) causes.
- Both positive and negative feedbacks are covered
- The unintuitive nature of correlation [Me: the only time math is used in the book. It’s used lightly but critically. Anyone running a relative value book will appreciate the demonstrations of non-linearity]
Â
Chapter 9: Alignment
Rule: Time spent on alignment is time well-spent
This is a must-read chapter for any manager.
- See why Google’s “Don’t Be Evil” was a poor message for alignment.
- See the hidden options that employees and asset managers will ferret out [me: i’ve thought about alignment a lot over my career. I think it’s one of, if not, the hardest problems in finance. Moloch’s hands are all over compensation and fee issues]
- The author makes a simple yet powerful observation that too many people willfully ignore:
- True alignment can only happen by collapsing the distinction between capital (the people with the money) and labor (the people doing the work).
Â
Chapter 10: Technology
Rule: If you don’t master technology and data you’re losing to someone who does
- The importance of data and what types
- The role of human traders. What gives them their value and how big is that value. As automation increases, this discussion is going to be counterintuitive but applies to many fields. technology amplifies creative and decision-making leverage.
- Technical topics
- Software systems
- Debugging [me: this was an in-depth meta discussion esp interesting since I’m not a developer]
- Technical debt [me: seeing a trader tackle this topic was eye-opening; one of the longer discussions in the book]
Â
Chapter 11: Adaptation
Rule: If you’re not getting better, you’re getting worse
Â
A brilliant chapter recapping each of the rules and how they fit into the evolving nature of markets. The metaphor to biology is effective and tight. The bonus aspect of this chapter is how it tied the the entire book into a story of self-improvement on both individual and group levels. It includes framing transforms the burden of evolutionary pressures to opportunities.
Â
The trading contexts in this book are a thick skin on what is happening underneath.
It is actually adapting the lessons from markets and decision-making to explore the richness of life itself by animating our collaborative natures. It harnesses our privileged intellectual endowment in the hierarchy of nature in service of our inclinations towards human progress and flourishing.
Â
Â
Â
Â
Â
Â